目錄
- 一、準(zhǔn)備
- 二、三次樣條插值
- 三、最小二乘擬合
- 四、拉格朗日乘子法
一、準(zhǔn)備
噪聲是在擬合過程中常用的干擾手段,常用的噪聲:
1.統(tǒng)一分布 U(a,b)
f ( x ) = { 1 i f a ≤ x b 0 o t h e r f(x)=\begin{cases}\begin{aligned}1\quad if\quad a\le xb \\ 0\quad other\end{aligned}\end{cases} f(x)={10ifa≤xbother
import numpy as np
x=np.random.uniform(a,b,100) #產(chǎn)生長度為100的U(a,b)
2.正態(tài)分布N( μ \mu μ, σ 2 \sigma^2 σ2)
import numpy as np
x=np.random.normal(mu, sig, 100) #產(chǎn)生長度為100的N(mu, sqart(sig))
二、三次樣條插值
def spline_fit():
size = 20
x = np.linspace(-10, 10, size)
y = np.sin(x) + np.random.normal(0, 0.1, size)
y2 = [0] * len(y)
# for y_i in y:
pp.plot(x, y)
cs = CubicSpline(x, y)
x2 = x = np.linspace(-10, 10, size * 100)
pp.plot(x2, cs(x2))
pp.show()
三、最小二乘擬合
def least_square():
f = lambda p0, xx: p0[0] * np.sin(xx * p0[1]) + p0[2]
LEN = 100
x = np.linspace(-1, 1, LEN)
y = x ** 2 + 5
# 默認(rèn)情況,param只會(huì)返回求得的參數(shù)和返回的錯(cuò)誤碼,1-4為成功,5-8為失敗,如果想輸出更多參數(shù),可以指定full_out=1,可以看到出錯(cuò)原因和其他參數(shù)
param = leastsq(lambda p0, xx, yy: f(p0, xx) - yy, (1, 1, 1), args=(x, y)) #初值的選擇比較重要,如果選取不當(dāng),容易陷入局部最優(yōu)
print(param)
pp.scatter(x, y)
p0 = param[0]
pp.plot(x, f(p0, x))
pp.show()
最小二乘的初值選取非常重要,以下是三份完全相同的數(shù)據(jù),雖然最后都收斂了,但是初值不同,得到了完全不同的擬合結(jié)果
初值為 ( 1 , 2 , 1 ) (1,2,1) (1,2,1)
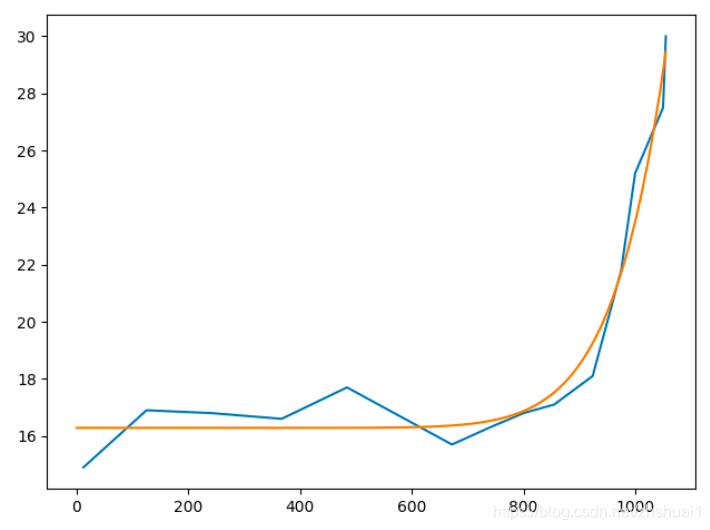
初值為 ( 1 , 1 , 1 ) (1,1,1) (1,1,1)

初值為 ( 10 , 10 , 1 ) (10,10,1) (10,10,1)
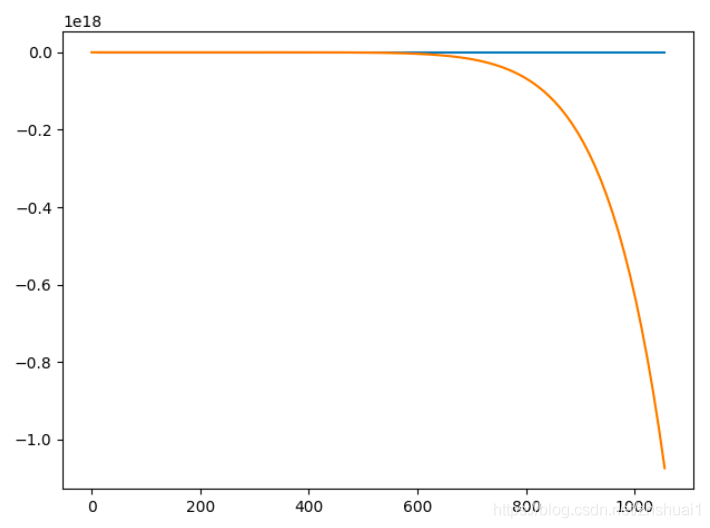
四、拉格朗日乘子法
def lagrange()
from scipy.optimize import minimize
import numpy as np
e = 1e-10
fun = lambda x: 8 * (x[0] * x[1] * x[2]) # f(x,y,z) =8 *x*y*z
cons = ({'type': 'eq', 'fun': lambda x: x[0] ** 2 + x[1] ** 2 + x[2] ** 2 - 1}, # x^2 + y^2 + z^2=1
{'type': 'ineq', 'fun': lambda x: x[0] - e}, # x>=e等價(jià)于 x > 0
{'type': 'ineq', 'fun': lambda x: x[1] - e},
{'type': 'ineq', 'fun': lambda x: x[2] - e}
)
x0 = np.array((1.0, 1.0, 1.0)) # 設(shè)置初始值
res = minimize(fun, x0, method='SLSQP', constraints=cons)
print('最大值:', res.fun)
print('最優(yōu)解:', res.x)
print('迭代終止是否成功:', res.success)
print('迭代終止原因:', res.message)
到此這篇關(guān)于教你如何利用python進(jìn)行數(shù)值分析的文章就介紹到這了,更多相關(guān)python數(shù)值分析內(nèi)容請搜索腳本之家以前的文章或繼續(xù)瀏覽下面的相關(guān)文章希望大家以后多多支持腳本之家!
您可能感興趣的文章:- python實(shí)現(xiàn)各種插值法(數(shù)值分析)
- python實(shí)現(xiàn)數(shù)值積分的Simpson方法實(shí)例分析
- python 解決微分方程的操作(數(shù)值解法)
- Python導(dǎo)入數(shù)值型Excel數(shù)據(jù)并生成矩陣操作
- Python實(shí)現(xiàn)列表中非負(fù)數(shù)保留,負(fù)數(shù)轉(zhuǎn)化為指定的數(shù)值方式
- 使用Python matplotlib作圖時(shí),設(shè)置橫縱坐標(biāo)軸數(shù)值以百分比(%)顯示
- Python如何將函數(shù)值賦給變量