有時候我們會有這樣的想法,就是針對某個區(qū)域的柵格數(shù)據(jù),要提取它的平均值或者其他統(tǒng)計指標(biāo),比如在一個省內(nèi)提取多年的降雨數(shù)據(jù),最后分區(qū)域地計算一些統(tǒng)計值,或者從多個柵格數(shù)據(jù)中提取某個區(qū)域的數(shù)值形成一個序列。為了方便,畫一個示意圖看看,比如就像提取這個區(qū)域中的某一個市的區(qū)域,然后形成一個序列數(shù)據(jù),這就可以使用rasterstats庫了,此外的分區(qū)統(tǒng)計也可以用這個庫
這個實驗使用的數(shù)據(jù)格式分別是柵格(*.tif)和矢量(.shp),之后的分區(qū)統(tǒng)計操作和柵格數(shù)據(jù)的提取都是源于這兩類數(shù)據(jù)。為了能使用上這個rasterstats庫,選擇了在google colab平臺運行腳本,因為安裝庫實在是太方便了,在win上老是安裝不上的,在google notebook立馬就搞定了,而且可以把數(shù)據(jù)存儲到谷歌云盤,直接在notebook中就是可以鏈接使用的
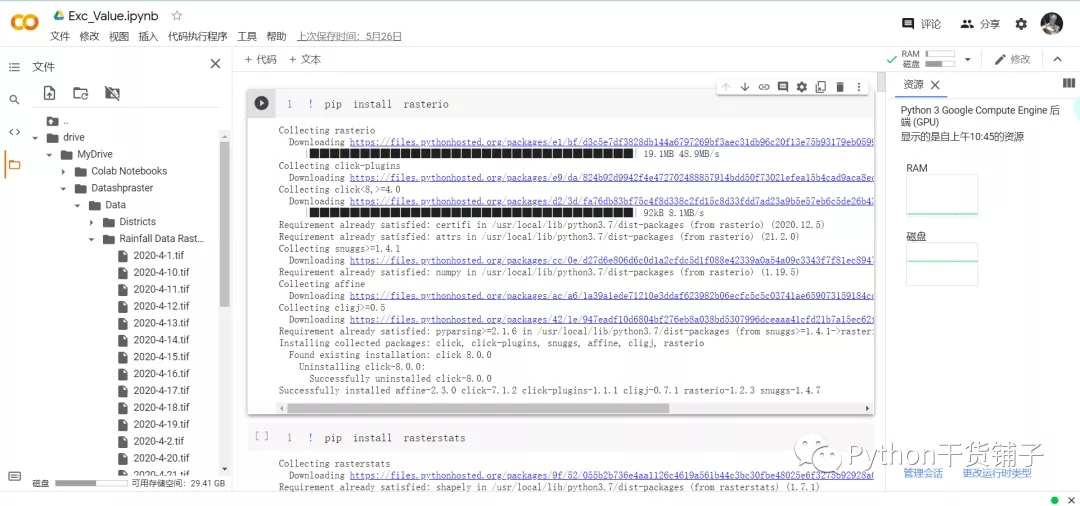
那么現(xiàn)在就開始做測試,使用的數(shù)據(jù)就是左側(cè)的柵格和矢量數(shù)據(jù)集
導(dǎo)入相關(guān)的模塊
import geopandas as gpd
import pandas as pd
import numpy as np
import matplotlib.pyplot as plt
import rasterio
import rasterstats
from rasterio.plot import show
# show()方法用來展示柵格圖形
from rasterio.plot import show_hist
# 用來展示直方圖
import cartopy.crs as ccrs
import cartopy.feature as cfeature
from cartopy.mpl.ticker import LongitudeFormatter, LatitudeFormatter
使用geopandas和rasterio分別讀取矢量和柵格數(shù)據(jù)
# 使用geopandas讀取矢量數(shù)據(jù)
districts = gpd.read_file('/content/drive/MyDrive/Datashpraster/Data/Districts/districts.shp')
# 使用rasterio讀取柵格數(shù)據(jù),柵格數(shù)據(jù)和矢量數(shù)據(jù)的坐標(biāo)投影需要一致
raster = rasterio.open('/content/drive/MyDrive/Datashpraster/Data/Rainfall Data Rasters/2020-4-1.tif')
# 把矢量數(shù)據(jù)和柵格數(shù)據(jù)繪制到一個axis上,這個axis不是坐標(biāo)軸,而是圖形
plt.rcParams['font.family'] = 'Times New Roman'
plt.rcParams['font.size'] = 20
fig, (ax1,ax2) = plt.subplots(1,2,figsize=(15,6))
show(raster, ax=ax1,title='Rainfall')
# 讀取進(jìn)來的矢量數(shù)據(jù)可以直接調(diào)用gpd的plot()方法繪制
districts.plot(ax=ax1, facecolor='None', edgecolor='red')
show_hist(raster,ax=ax2,title='hist')
plt.show()
先繪制一下結(jié)果看看
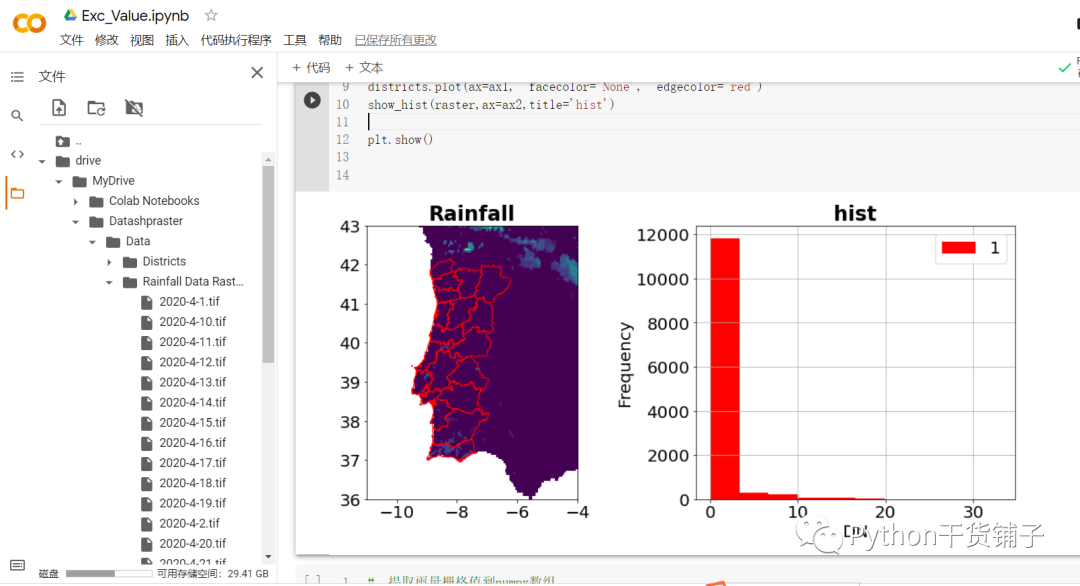
讀取柵格數(shù)據(jù):
# 提取雨量柵格值到numpy數(shù)組
# 遵循GDAL規(guī)則從第一波段讀取
rainfall_data = raster.read(1)
rainfall_data
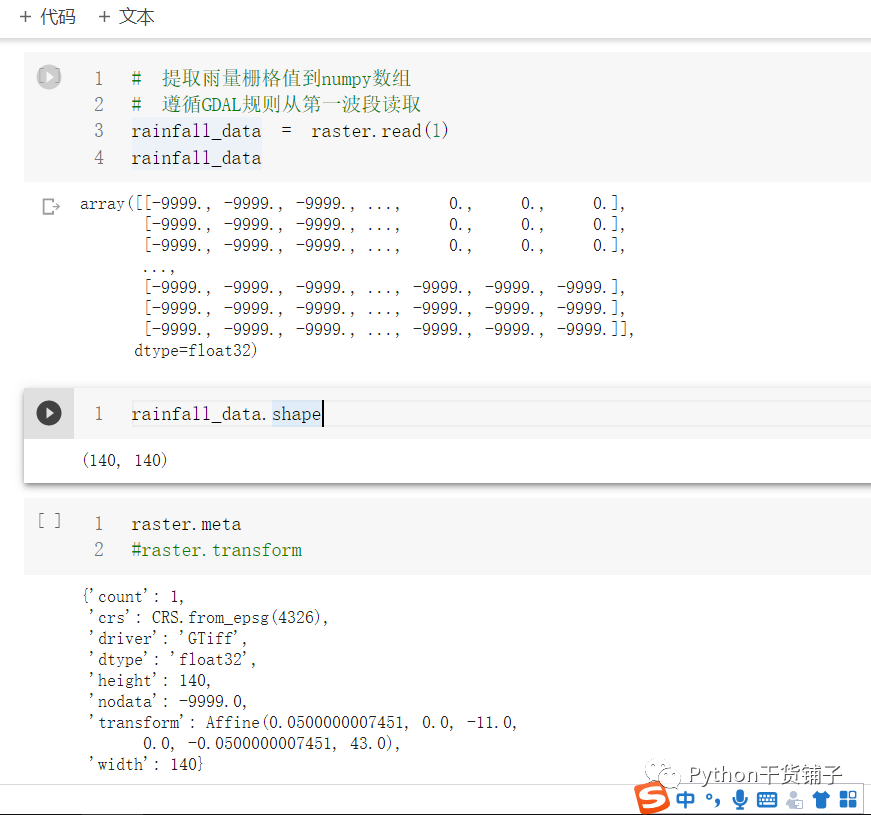
開始分區(qū)統(tǒng)計:
# 設(shè)置坐標(biāo)變換信息
affine = raster.transform
# 準(zhǔn)備開始進(jìn)行空間分區(qū)計算
# 第一個參數(shù)是矢量分區(qū),第二個是柵格,第三個是坐標(biāo)變換信息,第四個是統(tǒng)計均值
avg_rallrain = rasterstats.zonal_stats(districts,rainfall_data,affine=affine,stats=['mean'],geojson_out=True)
# avg_rallrain
# 除了統(tǒng)計平均值之外,還有最大最小值那些
繪制一下,只是一個簡單的圖形而已
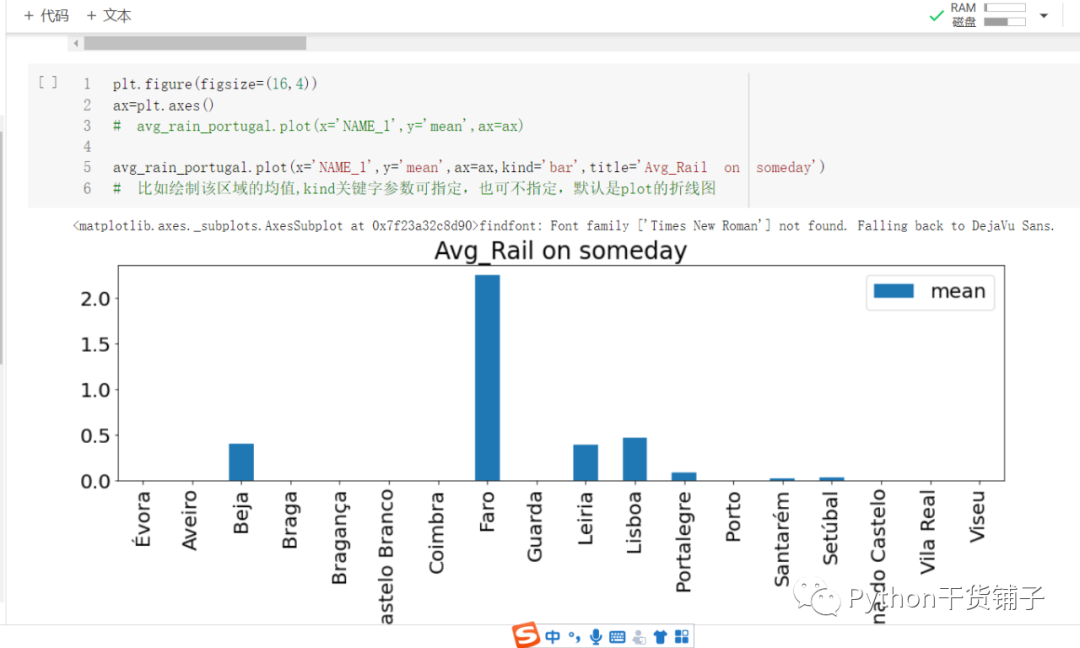
當(dāng)然第二部分更有意思,就是從多個分散的柵格數(shù)據(jù)中提取數(shù)據(jù)形成一個序列
,就是這些tif數(shù)據(jù)
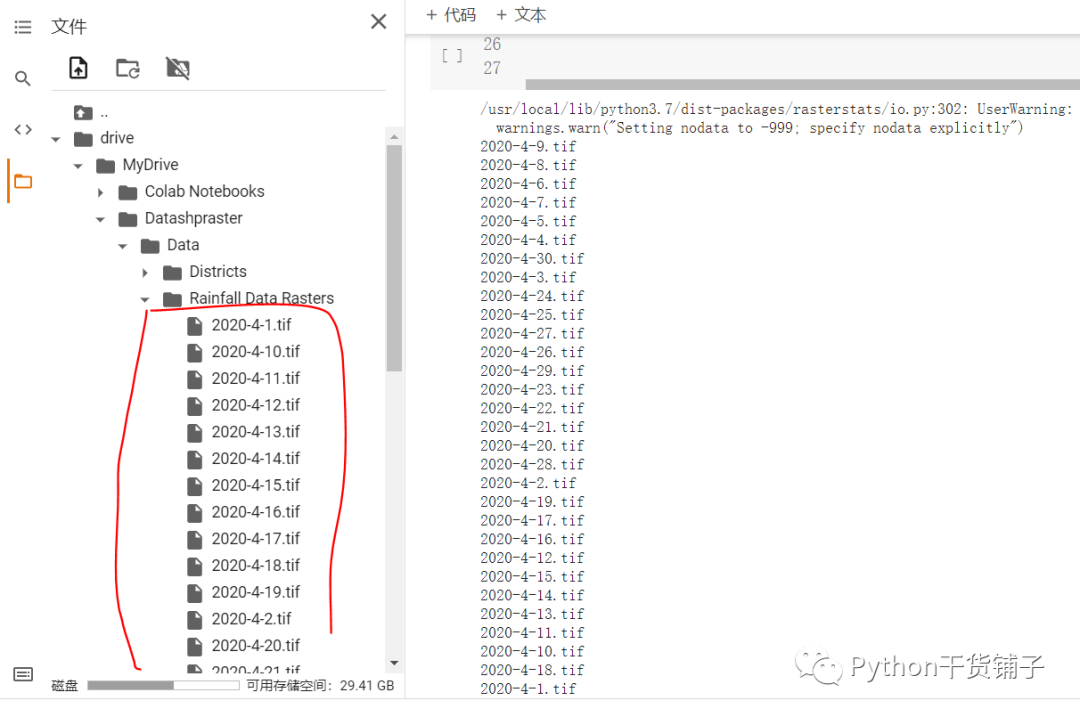
loop這些柵格數(shù)據(jù)集:
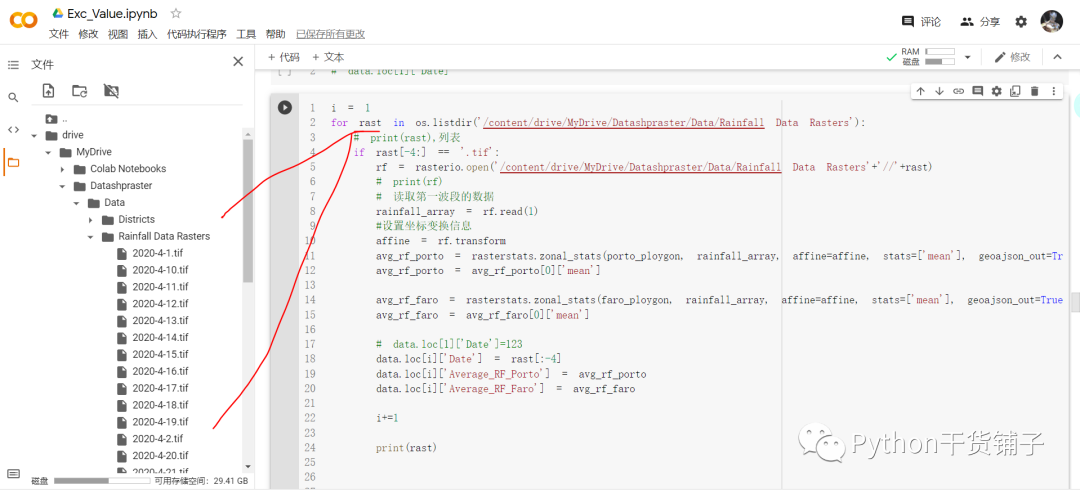
獲得提取到的結(jié)果,沒錯,就是這么一個序列數(shù)據(jù),然后就是繪圖了
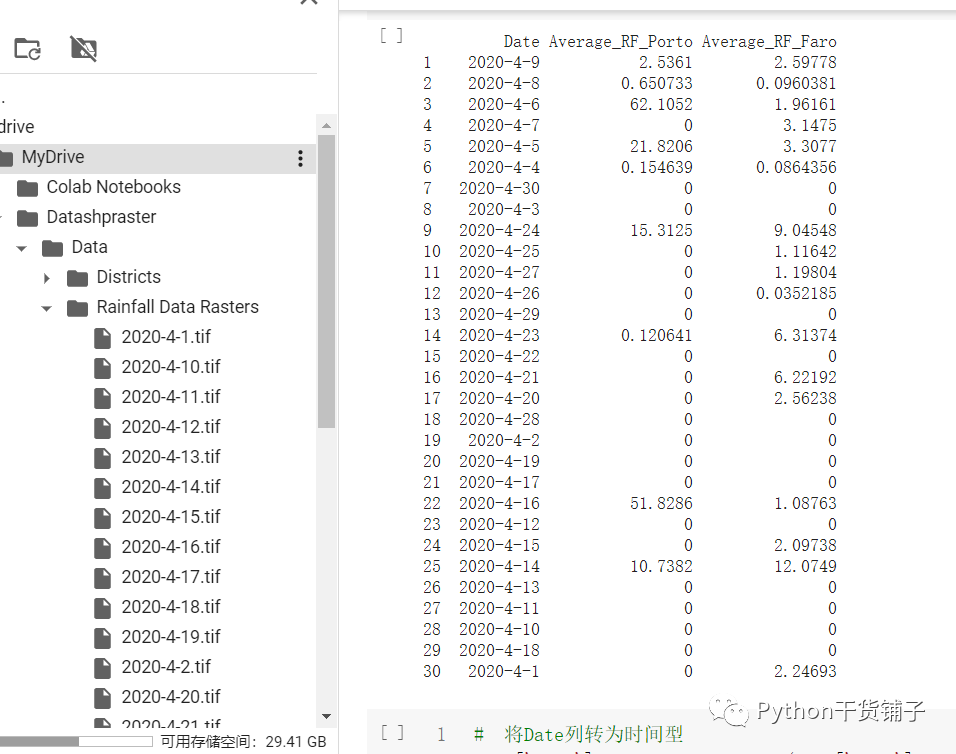
轉(zhuǎn)換數(shù)據(jù)格式
# 將Date列轉(zhuǎn)為時間型
data['Date'] = pd.to_datetime(data['Date'], infer_datetime_format=True)
# print(data)
data['Date'] = data['Date'].dt.date
print(data)
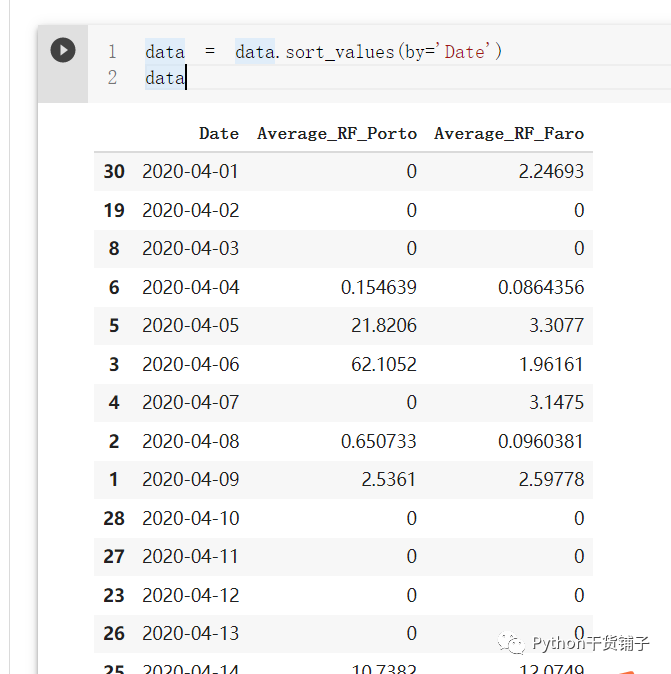
繪圖結(jié)果就是簡單的圖形而已
# 準(zhǔn)備繪制圖形
fig,(ax1,ax2)= plt.subplots(2,1,figsize=(18,6))
plt.rcParams['font.size'] = 15
data.plot(x='Date', y='Average_RF_Porto', ax=ax1, kind='bar', title='Avg_Rail_Porto')
data.plot(x='Date', y='Average_RF_Faro', ax=ax2, kind='bar', title='Avg_Rail_Faro',color='red')
#自動調(diào)整圖形的分布
plt.tight_layout()
plt.show()
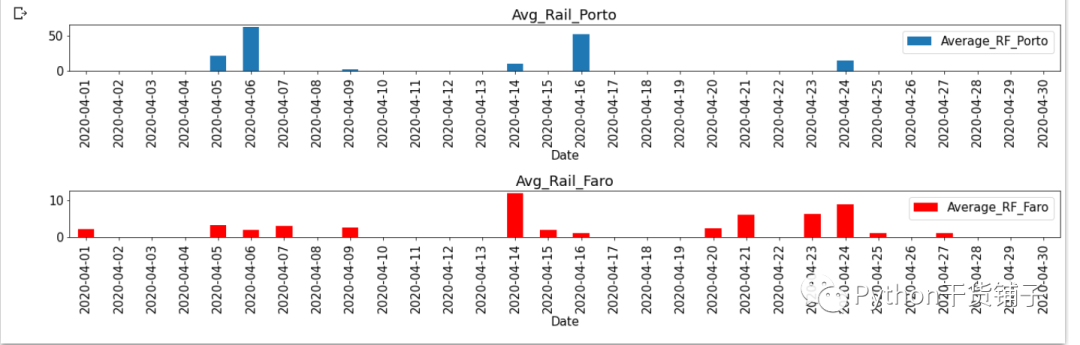
結(jié)果就這樣一個序列圖,目的就是從柵格提取指定的研究區(qū),然后提取柵格的值,再來繪圖
雖然感覺不是那么花里胡哨的圖,但這個應(yīng)該還是比較實用的,特別是大批量提取柵格值的時候。由于在google colab里面操作的步驟比較多,中間可能有省略的地方,但重要的應(yīng)該都在文中了,當(dāng)然也可以遷移運用到其他地方,也可以查看一下這個第三方庫的教程,比如read(1)是什么意思,官網(wǎng)的docs就寫得有,實在是很方便的
以上就是用Python進(jìn)行柵格數(shù)據(jù)的分區(qū)統(tǒng)計和批量提取的詳細(xì)內(nèi)容,更多關(guān)于Python 柵格數(shù)據(jù)的分區(qū)統(tǒng)計和批量提取 的資料請關(guān)注腳本之家其它相關(guān)文章!
您可能感興趣的文章:- 在Python中用GDAL實現(xiàn)矢量對柵格的切割實例
- python獲取柵格點和面值的實現(xiàn)
- python 矢量數(shù)據(jù)轉(zhuǎn)柵格數(shù)據(jù)代碼實例
- 關(guān)于Python 的簡單柵格圖像邊界提取方法
- Python疊加兩幅柵格圖像的實現(xiàn)方法